With the fast paced evolution of Fintech Ecosystem in India in the last few years, identity theft has become the largest contributor to fraud. With so much data being leaked online, it’s astonishingly easy for fraudsters to mimic another individuals identity, use stolen credit card information or take over an account. Juniper Research estimates that the global cost of data breaches will rise to $2.1 trillion by 2019. As per Firstpost, India alone accounted for 37 percent of the world’s data breaches in 20182. An all-digital world amplifies the classic threats of service misuse, identity impersonation and money laundering. Businesses want to offer quick, convenient access. But they also want to keep fraudulent users off their platform. Users worry too, about keeping their identity information secure. And rightly so—the current state of play is fragmented and out of date. Identity information should not be this easily duplicated or poorly stored. Data breaches should be the exception, not the norm. Verifying a person’s true identity should be the key to managing risk.
In the past, we’ve seen a few different approaches to verify an online user’s identity. Databases, government ID documents, phone numbers, emails, social networks, biometrics, etc. Geographical reach, user experience and fraud prevention exist in a balance. Reliable approaches to verification will use these methods in some combination to properly assess the identity being claimed. Lower assurance models can be used as weaker signals, or in first-pass cascade for a layered authentication. An e-book released by Onfido’s CPO, Kevin Trilli shows the below table which gives a side-by-side comparison of each approach to identity verification.
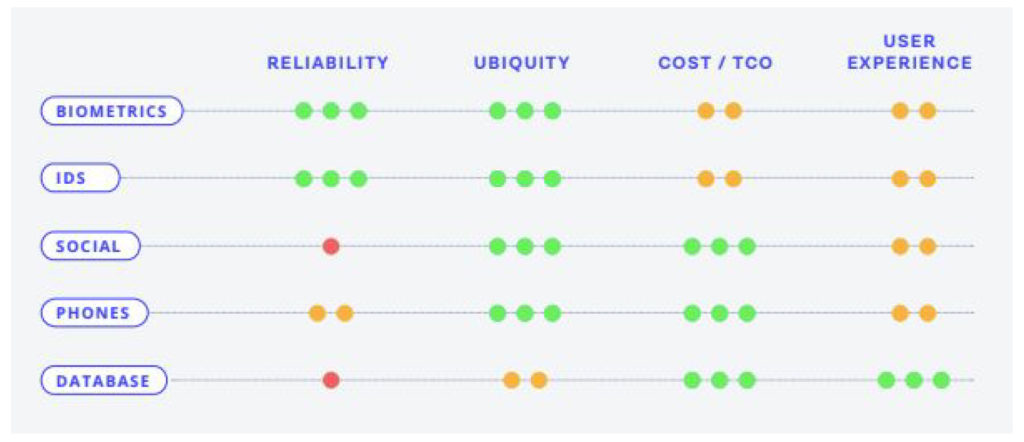
Fraudulent identities come in many varieties. Fraudsters can illegally change information on the Document Id so that they can modify part or all of the identity. They can change the variable information, inserting real segments from another ID, remove parts or specific information, applying false stamps or watermarks or digitally alter or add information on an image of an original document ID. Below are some of the most common examples of Fraudulent Aadhaar Cards in India.
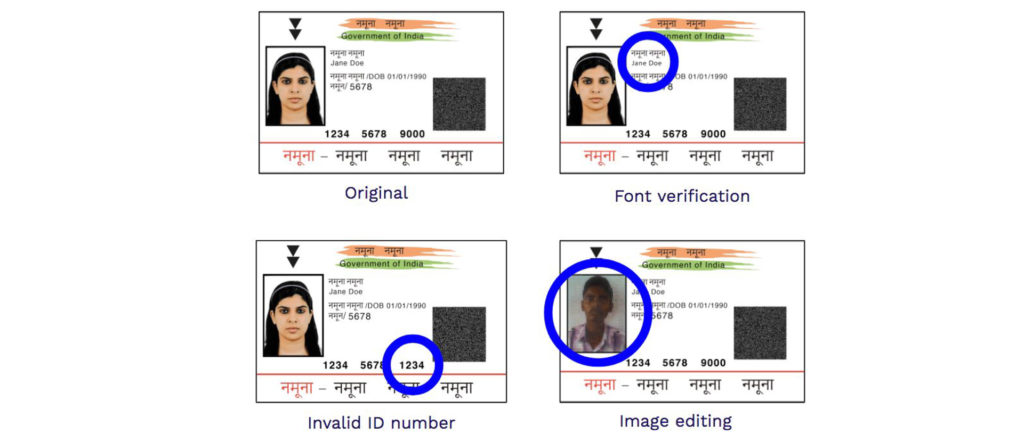
Each of these Document IDs shows fraudulent characteristics, requiring different detection methods. These specific detection techniques ensure that the ID hasn’t been modified, that it’s a legitimate ID and that it belongs to the person presenting it. For every fraud technique, there’s a corresponding detection technique. For example, data consistency is a detection technique built to protect against fraudsters tampering with the text in the document. Similarly, photo swap detection is a technique built to protect against fraudsters swapping the original photo in the document with a false one. Many IDs have specific design and layout templates, and built-in security features. Document template comparison can identify these errors or inconsistencies against the standard document template.
Now that we have confirmed that the ID is authentic, how would we make sure that the user presenting the ID is its authorized owner? To prove ownership, we can compare a picture of the user with the ID photo to confirm facial similarity. However, fraudulent users can attempt to spoof the system with a printout of the target’s face, or photos from their social media profile, or even sophisticated 3D masks. Online verification flows, along with algorithmic comparison techniques, can protect against these attack vectors. We can minimize the risk of photos from other sources being used by ensuring that the image or video submitted was created on the user’s device, and detecting the time the photo or video was created. Live selfie photos protect against common spoofing attempts, like providing a photo of a photo, by using passive anti-spoofing algorithms. Video liveness provides the highest level of assurance against sophisticated spoofing attempts, including deep fakes. As instead of taking a selfie, the user films himself reading out numbers and performing randomized movements. This proves liveness in a “challenge and response” interaction.
However, doing this at high volume globally is tough. A human-only approach can’t deliver accurate results at scale. Enter the machine learning approach which uses data to train the model and delivers results in lighting fast speed. But are they clear when to escalate the human judgement? What if they get new formats of documents that they have not been trained for? They will fail the document even though it was a real one. What is the solution then? The answer is Supervised Machine Learning Approach. A technique designed to mimic the way a human brain works, for the broadest and most effective performance. A hybrid combination of both machine learning and expertise human intelligence. This would help catch the highest degree of possible fakes along with reducing the drop-offs. This is exactly the approach we have adopted at Onfido. Our identity verification (IDV) platform includes a risk engine that combines various signals to assess whether a user is in fact the person they claim to be. It leverages human oversight and machine learning combined with advanced IDV technologies to deliver powerful and effective fraud detection.
To choose an identity verification process, all approaches in the comparison matrix should be considered and the approach that best fits the business’ risk profile, speed thresholds and user experience goals, should be selected. The low reliability of databases, devices and social networks means that they should always be used in combination with IDs or biometrics to fully assess the identity of risk of a person online. Let users use the most commonly available forms of legal identity as proof. Let companies get true assurance that users are who they claim to be by combining users’ legal identities with biometric analysis.
Disclaimer: The opinions expressed within this article are the personal opinions of the author. The facts and opinions appearing in the article do not reflect the views of DLAI and DLAI does not assume any responsibility or liability for the same